Automatic emotion recognition
Emotions affect our perception, reasoning, and decision-making in many different ways, but they can also be used to detect the state of the mind of some individuals. Similarly, human-machine interaction would be more intuitive and smooth if we incorporate emotional intelligence into machines. This motivated researchers to develop algorithms that could be used for automatic detection of individuals’ emotional states, mostly deep learning (DL), but this task is far from trivial for several reasons.
First, there is no unique classification of emotions. The attempts to classify emotions could be divided into two groups. The first one describes the emotional space with a finite number of prototypical emotions. One of the most prominent representative theories from this group states there are six basic emotions: happiness, sadness, disgust, fear, surprise, and anger. There are some other theories arguing this number is higher. The second group of approaches uses dimensions to describe emotion space, typically arousal (describing pleasantness in the range from positive to negative) and valence (describing activation during emotion expression from passive to active).
Additional problem in automatic emotion recognition (AER) is represented in the fact that people express their emotions through different modalities such as visual, auditory, physiological, and olfactory. The choice of words could also be changed. Also, when people perceive emotions by the other people clues from couple of different modalities are used. Additionally, as in many other machine learning tasks, there is often a mismatch between preprocessed database used for model training and real-life conditions.
It is expected that AER will continue to be applied in many different areas such as security, medical diagnosis, patient care, autonomous driving, entertainment, education, and public services. In some call centers, it is already used to detect the emotional state of customers and to adjust actions accordingly. Some movie studios use this technology to track the audience’s reaction during test projections. It can be implemented to track students’ emotions during online courses and give lecturers feedback about the quality of their classes or help doctors to detect depression and dementia.
Within the MARVEL project, we are dealing with audio-visual emotion recognition. As most of the available databases are recorded by professionals, one of our major goals is to collect a database of amateur speakers, which should further provide more precise DL models as the amateur reactions are more natural. It should be noted that all volunteers use their own phones so that there is a variety of cameras used for recordings. For the purposes of database creation, a dedicated Android application is developed. The volunteers perform the recording by listening to the reference recording of the same utterance, recorded by a professional actor, before proceeding with recording their own rendition of the utterance. The application workflow requires the user to listen and watch his/her recording of the utterance at least once before proceeding to the next utterance. The users are also allowed to re-record their own rendition of the utterance as many times as they wish, and the previous recording is immediately discarded. The current recording process is performed in the Serbian language, although the app could be adjusted to some other languages.
References
-
[1]
Blog signed by: the UNS team
Menu
- Home
- About
- Experimentation
- Knowledge Hub
- ContactResults
- News & Events
- Contact
Funding
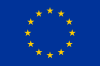
This project has received funding from the European Union’s Horizon 2020 Research and Innovation program under grant agreement No 957337. The website reflects only the view of the author(s) and the Commission is not responsible for any use that may be made of the information it contains.