Detailed info
Communication efficient model-aware federated learning for visual crowd counting and density estimation in smart cities
Authors | A. Armacki, N. Milosevic, D. Bajovic, S. Kar, D. Jakovetic, A. Bakhtiarnia, L. Esterle, A. Muscat, and T. Festi |
Title | Communication efficient model-aware federated learning for visual crowd counting and density estimation in smart cities |
Abstract | Federated learning (FL) is an attractive paradigm where a number of users can improve their local models via sharing trained models or model increments with a central server, while the users’ data is kept private. However, when model sizes are huge, FL incurs a significant communication overhead. In such scenarios, strategies that perform user sampling, so that only informative users communicate their models to the server, are desired. In this paper, we make several contributions on user sampling in FL. On the theoretical side, we consider a general framework that exhibits user heterogeneity across several dimensions: activation probabilities, gradient noise variance, number of updates per epoch, and communication channel quality. In this setting, we derive convergence rate of the FedAvg method. The rate explicitly characterizes the effects of heterogeneity and enables us to derive optimal user sampling probabilities in an offline setting, when the sampling probabilities are pre-computed. We then show how these derived probabilities naturally connect with existing optimized sampling strategies in an adaptive-online setting. On the practical side, we study visual crowd counting (VCC) as a representative deep learning application with huge-sized models. We provide an implementation of the FL system over real-world data across three pilot sites – Valetta, Trento and Novi Sad. The evaluation results demonstrate significant model accuracy benefits through employing FL over the multiple cities, and significant communication savings via non-uniform user sampling strategies. |
Conference | 31st European Signal Processing Conference (EUSIPCO 2023) |
Date | 04-08 September 2023 |
Location | Helsinki, Finland |
Year of Publication | 2023 |
Url | https://zenodo.org/records/10628909 |
DOI | 10.23919/EUSIPCO58844.2023.10289938 |
Menu
- Home
- About
- Experimentation
- Knowledge Hub
- ContactResults
- News & Events
- Contact
Funding
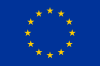
This project has received funding from the European Union’s Horizon 2020 Research and Innovation program under grant agreement No 957337. The website reflects only the view of the author(s) and the Commission is not responsible for any use that may be made of the information it contains.