Detailed info
ConfMix: Unsupervised Domain Adaptation for Object Detection via Confidence-based Mixing
Authors | Giulio Mattolin, Luca Zanella, Elisa Ricci, Yiming Wang |
Title | ConfMix: Unsupervised Domain Adaptation for Object Detection via Confidence-based Mixing |
Abstract | Unsupervised Domain Adaptation (UDA) for object detection aims to adapt a model trained on a source domain to detect instances from a new target domain for which annotations are not available. Different from traditional approaches, we propose ConfMix, the first method that introduces a sample mixing strategy based on region-level detec- tion confidence for adaptive object detector learning. We mix the local region of the target sample that corresponds to the most confident pseudo detections with a source image, and apply an additional consistency loss term to gradually adapt towards the target data distribution. In order to robustly define a confidence score for a region, we exploit the confidence score per pseudo detection that accounts for both the detector-dependent confidence and the bounding box uncertainty. Moreover, we propose a novel pseudo la- belling scheme that progressively filters the pseudo target detections using the confidence metric that varies from a loose to strict manner along the training. We perform ex- tensive experiments with three datasets, achieving state-of-the-art performance in two of them and approaching the supervised target model performance in the other. |
ISBN | TBA |
Conference | IEEE/CVF Winter Conference on Applications of Computer Vision (WACV), 2023 |
Date | 03-07/01/2023 |
Location | Waikoloa, Hawaii, USA |
Year of Publication | 2023 |
Url | https://zenodo.org/record/7330908 |
DOI | TBA |
Menu
- Home
- About
- Experimentation
- Knowledge Hub
- ContactResults
- News & Events
- Contact
Funding
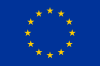
This project has received funding from the European Union’s Horizon 2020 Research and Innovation program under grant agreement No 957337. The website reflects only the view of the author(s) and the Commission is not responsible for any use that may be made of the information it contains.