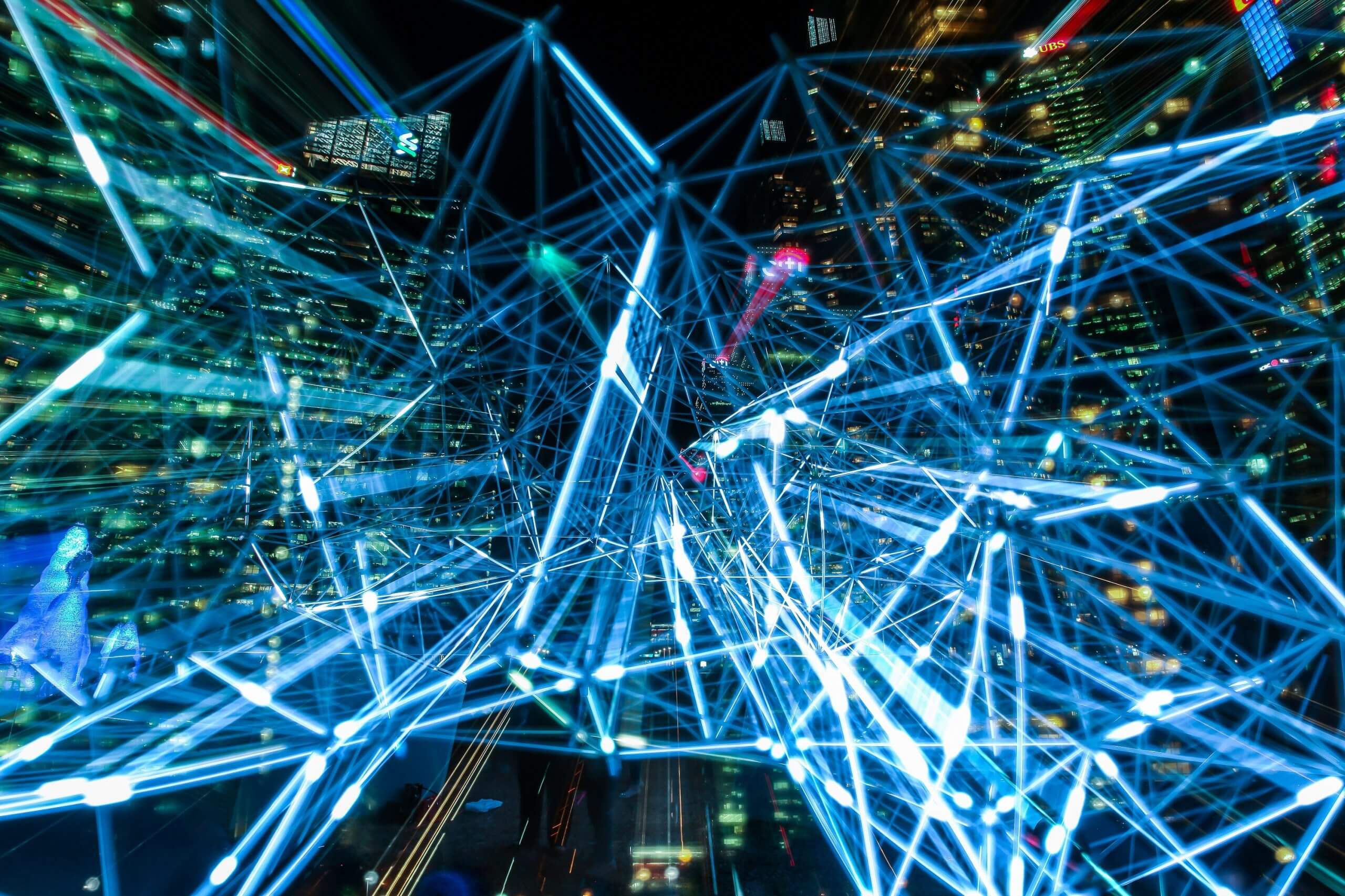
Data Privacy and data protection
Data privacy and data protection is particularly important given the sensitivity of society and the impac on the project on it. In terms of safeguarding privacy and offering better data protection, MARVEL goes beyond the State of the Art by utilizing the advanced federated learning. With federated learning, DL training mechanism among the end, the edge and the cloud-edge devices can train their local DL models with their own collected data and upload only the updated model in the cloud. Further, the secure aggregation and differential privacy can be applied in this context. These techniques represent additional safeguards which are useful in avoiding the disclosure of sensitive information including personal data contained in local updates
GPU Stream Processing in edge devices and in the cloud
MARVEL will build on current trends and dynamically assign initial execution parts to the edge device’s GPU and then forward the remaining to the cloud’s GPU pool. The pattern matching engine will be evaluated in edge devices, measuring the throughput of the incoming processed data, as well as their power consumption. To evaluate the performance of the pattern matching engine in cloud environments, the throughput using streaming data and multiple GPU devices will be measured, and the system’s ability to schedule the incoming data streams to the corresponding GPU devices for efficiency purposes will be evaluated.
Edge Node Security
MARVEL will explore trusted execution environments, which can enable remote attestation and further provide full memory encryption and will revisit the existing and well-known system hardening techniques, and we will re-design and adapt them to the requirements of IoT devices according to their hardware capabilities, in order to protect them from advanced code injection and modification attacks. Edge node security evaluation will be based on measurements of the effectiveness and responsiveness of the system, as well as of the performance overhead that it adds to the system. Finally, we will explore novel programming paradigms tailored for IoT, that will enable the robust, efficient, and trusted data flow control to externally-paired field gateways. By doing so, the IoT devices will securely offload data storage and computations in a trustworthy manner, without necessarily being equipped with TEE-enabled CPUs
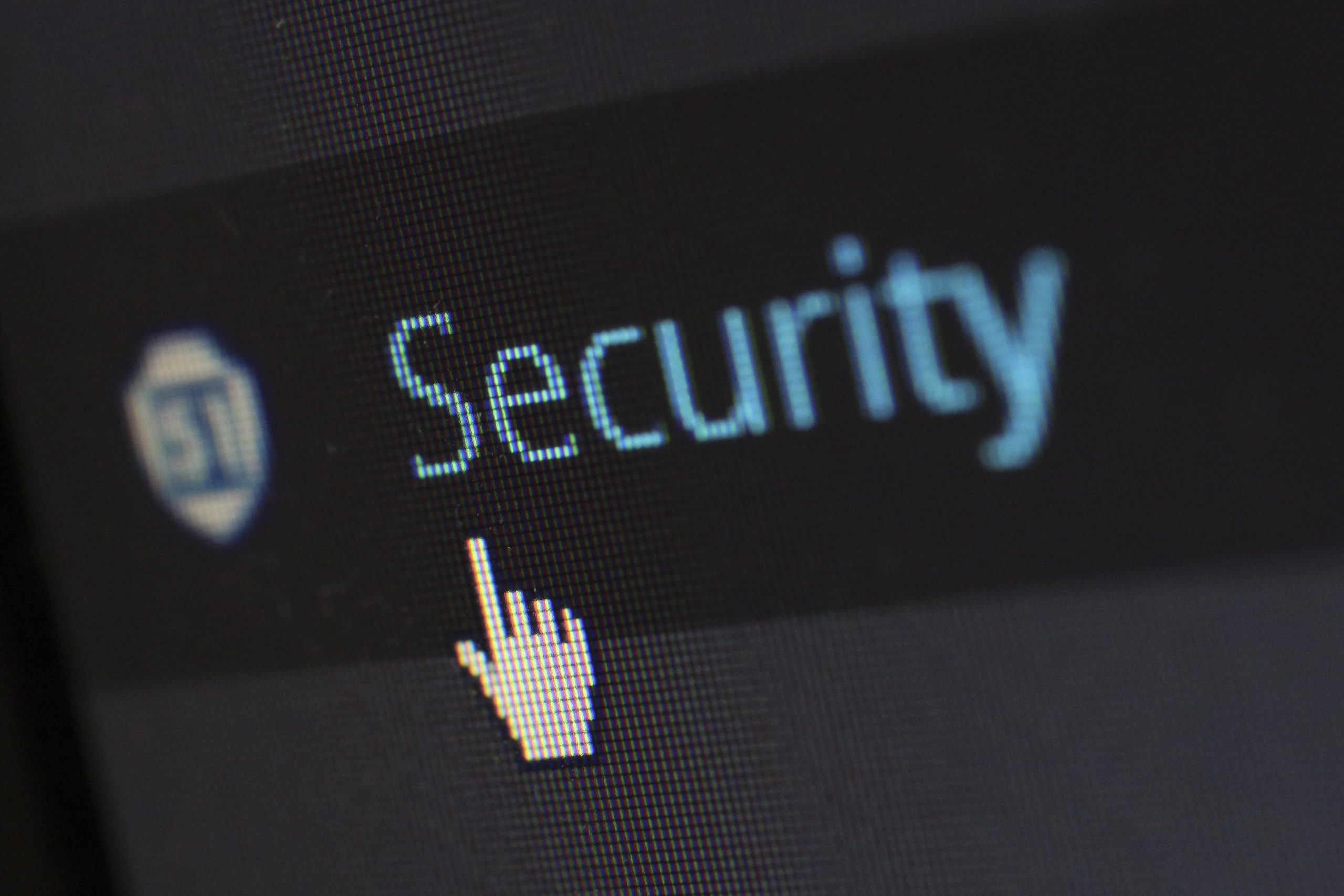
Funding
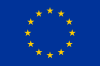
This project has received funding from the European Union’s Horizon 2020 Research and Innovation program under grant agreement No 957337. The website reflects only the view of the author(s) and the Commission is not responsible for any use that may be made of the information it contains.