Detailed Info
Efficient High-Resolution Deep Learning: A Survey
Authors: | Arian Bakhtiarnia, Qi Zhang, & Alexandros Iosifidis |
Title: | Efficient High-Resolution Deep Learning: A Survey |
Abstract: | Cameras in modern devices such as smartphones, satellites and medical equipment are capable of capturing very high-resolution images and videos. Such high-resolution data often need to be processed by deep learning models for cancer detection, automated road navigation, weather prediction, surveillance, optimizing agricultural processes and many other applications. Using high-resolution images and videos as direct inputs for deep learning models creates many challenges due to their high number of parameters, computation cost, inference latency and GPU memory consumption. Simple approaches such as resizing the images to a lower resolution are common in the literature, however, they typically significantly decrease accuracy. Several works in the literature propose better alternatives in order to deal with the challenges of high-resolution data and improve accuracy and speed while complying with hardware limitations and time restrictions. This survey describes such efficient high-resolution deep learning methods, summarizes real-world applications of high-resolution deep learning, and pro- vides comprehensive information about available high-resolution datasets. |
Publication type: | Journal |
Title of the journal: | |
Year of Publication | 2024 |
Pages: | TBD |
Number, date or frequency of the Journal: | February 2024 |
Publisher: | ACM |
URL: | https://zenodo.org/records/10629239 |
DOI | 10.1145/3645107 |
Menu
- Home
- About
- Experimentation
- Knowledge Hub
- ContactResults
- News & Events
- Contact
Funding
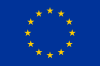
This project has received funding from the European Union’s Horizon 2020 Research and Innovation program under grant agreement No 957337. The website reflects only the view of the author(s) and the Commission is not responsible for any use that may be made of the information it contains.