Detailed info
XiNet: Efficient Neural Networks for tinyML
Authors | Alberto Ancilotto, Francesco Paissan, Elisabetta Farella |
Title | XiNet: Efficient Neural Networks for tinyML |
Abstract | The recent interest in the edge-to-cloud continuum paradigm has emphasized the need for simple and scalable architectures to deliver optimal performance on computationally constrained devices. However, resource-efficient neural networks usually optimize for parameter count and thus use operators such as depthwise convolutions, which do not maximally exploit the efficiency of resource-constrained devices. In this article, we propose XiNet, a novel convolutional neural architecture that targets edge devices. We derived the XiNet architecture from an extensive real-world efficiency analysis of various neural network operators (e.g., standard, depthwise, and pointwise convolutions). Compared to other mobile architectures, our approach substantially improves the performance-complexity trade-off by optimizing the number of operations, parameters, and working memory (RAM). Moreover, we show how XiNet can be easily adapted to different devices thanks to Hardware Aware Scaling (HAS), which enables disjoint optimization of RAM, FLASH, and operations count. We analyze the scaling properties of our architecture under different hardware constraints and validate it on the image classification task. Finally, we evaluate the performance of XiNet for object detection on the MS-COCO and VOC-2012 benchmarks and compare it with state-of-the-art mobile neural networks, achieving a 70% reduction in energy requirements with similar performance. |
Conference | IEEE/CVF International Conference on Computer Vision (ICCV) |
Date | 02-06, October 2023 |
Location | Paris, France |
Year of Publication | 2023 |
Url | https://openaccess.thecvf.com/content/ICCV2023/html/Ancilotto_XiNet_Efficient_Neural_Networks_for_tinyML_ICCV_2023_paper.html |
DOI | 10.5281/zenodo.10629941 |
Menu
- Home
- About
- Experimentation
- Knowledge Hub
- ContactResults
- News & Events
- Contact
Funding
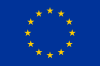
This project has received funding from the European Union’s Horizon 2020 Research and Innovation program under grant agreement No 957337. The website reflects only the view of the author(s) and the Commission is not responsible for any use that may be made of the information it contains.